mirror of
https://github.com/bitcoin/bitcoin.git
synced 2025-02-06 10:18:44 -05:00
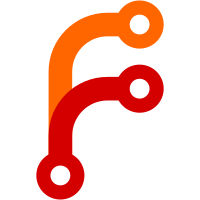
05300c1439
Use SelectionResult in SelectCoins (Andrew Chow)9d9b101d20
Use SelectionResult in AttemptSelection (Andrew Chow)bb50850a44
Use SelectionResult for waste calculation (Andrew Chow)e8f7ae5eb3
Make an OutputGroup for preset inputs (Andrew Chow)51a9c00b4d
Return SelectionResult from SelectCoinsSRD (Andrew Chow)0ef6184575
Return SelectionResult from KnapsackSolver (Andrew Chow)60d2ca72e3
Return SelectionResult from SelectCoinsBnB (Andrew Chow)a339add471
Make member variables of SelectionResult private (Andrew Chow)cbf0b9f4ff
scripted-diff: Use SelectionResult in coin selector tests (Andrew Chow)9d1d86da04
Introduce SelectionResult struct (Andrew Chow)94d851d28c
Fix bnb_search_test to use set equivalence for (Andrew Chow) Pull request description: Instead of returning a set of selected coins and their total value as separate items, encapsulate both of these, and other variables, into a new `SelectionResult` struct. This allows us to have all of the things relevant to a coin selection solution be in a single object. `SelectionResult` enables us to implement the waste calculation in a cleaner way. All of the coin selection functions (`SelectCoinsBnB`, `KnapsackSolver`, `AttemptSelection`, and `SelectCoins`) are changed to use a `SelectionResult` as the output parameter. Based on #22009 ACKs for top commit: laanwj: Code review ACK05300c1439
Tree-SHA512: e4dbb4d78a6cda9c237d230b19e7265591efac5a101a64e6970f0654e2c4f93d13bb5d07b98e8c7b8d37321753dbfc94c28c3a7810cb1c59b5bc29b08a8493ef
432 lines
18 KiB
C++
432 lines
18 KiB
C++
// Copyright (c) 2017-2020 The Bitcoin Core developers
|
||
// Distributed under the MIT software license, see the accompanying
|
||
// file COPYING or http://www.opensource.org/licenses/mit-license.php.
|
||
|
||
#include <wallet/coinselection.h>
|
||
|
||
#include <consensus/amount.h>
|
||
#include <policy/feerate.h>
|
||
#include <util/check.h>
|
||
#include <util/system.h>
|
||
#include <util/moneystr.h>
|
||
|
||
#include <numeric>
|
||
#include <optional>
|
||
|
||
// Descending order comparator
|
||
struct {
|
||
bool operator()(const OutputGroup& a, const OutputGroup& b) const
|
||
{
|
||
return a.GetSelectionAmount() > b.GetSelectionAmount();
|
||
}
|
||
} descending;
|
||
|
||
/*
|
||
* This is the Branch and Bound Coin Selection algorithm designed by Murch. It searches for an input
|
||
* set that can pay for the spending target and does not exceed the spending target by more than the
|
||
* cost of creating and spending a change output. The algorithm uses a depth-first search on a binary
|
||
* tree. In the binary tree, each node corresponds to the inclusion or the omission of a UTXO. UTXOs
|
||
* are sorted by their effective values and the tree is explored deterministically per the inclusion
|
||
* branch first. At each node, the algorithm checks whether the selection is within the target range.
|
||
* While the selection has not reached the target range, more UTXOs are included. When a selection's
|
||
* value exceeds the target range, the complete subtree deriving from this selection can be omitted.
|
||
* At that point, the last included UTXO is deselected and the corresponding omission branch explored
|
||
* instead. The search ends after the complete tree has been searched or after a limited number of tries.
|
||
*
|
||
* The search continues to search for better solutions after one solution has been found. The best
|
||
* solution is chosen by minimizing the waste metric. The waste metric is defined as the cost to
|
||
* spend the current inputs at the given fee rate minus the long term expected cost to spend the
|
||
* inputs, plus the amount by which the selection exceeds the spending target:
|
||
*
|
||
* waste = selectionTotal - target + inputs × (currentFeeRate - longTermFeeRate)
|
||
*
|
||
* The algorithm uses two additional optimizations. A lookahead keeps track of the total value of
|
||
* the unexplored UTXOs. A subtree is not explored if the lookahead indicates that the target range
|
||
* cannot be reached. Further, it is unnecessary to test equivalent combinations. This allows us
|
||
* to skip testing the inclusion of UTXOs that match the effective value and waste of an omitted
|
||
* predecessor.
|
||
*
|
||
* The Branch and Bound algorithm is described in detail in Murch's Master Thesis:
|
||
* https://murch.one/wp-content/uploads/2016/11/erhardt2016coinselection.pdf
|
||
*
|
||
* @param const std::vector<CInputCoin>& utxo_pool The set of UTXOs that we are choosing from.
|
||
* These UTXOs will be sorted in descending order by effective value and the CInputCoins'
|
||
* values are their effective values.
|
||
* @param const CAmount& selection_target This is the value that we want to select. It is the lower
|
||
* bound of the range.
|
||
* @param const CAmount& cost_of_change This is the cost of creating and spending a change output.
|
||
* This plus selection_target is the upper bound of the range.
|
||
* @returns The result of this coin selection algorithm, or std::nullopt
|
||
*/
|
||
|
||
static const size_t TOTAL_TRIES = 100000;
|
||
|
||
std::optional<SelectionResult> SelectCoinsBnB(std::vector<OutputGroup>& utxo_pool, const CAmount& selection_target, const CAmount& cost_of_change)
|
||
{
|
||
SelectionResult result(selection_target);
|
||
CAmount curr_value = 0;
|
||
|
||
std::vector<bool> curr_selection; // select the utxo at this index
|
||
curr_selection.reserve(utxo_pool.size());
|
||
|
||
// Calculate curr_available_value
|
||
CAmount curr_available_value = 0;
|
||
for (const OutputGroup& utxo : utxo_pool) {
|
||
// Assert that this utxo is not negative. It should never be negative, effective value calculation should have removed it
|
||
assert(utxo.GetSelectionAmount() > 0);
|
||
curr_available_value += utxo.GetSelectionAmount();
|
||
}
|
||
if (curr_available_value < selection_target) {
|
||
return std::nullopt;
|
||
}
|
||
|
||
// Sort the utxo_pool
|
||
std::sort(utxo_pool.begin(), utxo_pool.end(), descending);
|
||
|
||
CAmount curr_waste = 0;
|
||
std::vector<bool> best_selection;
|
||
CAmount best_waste = MAX_MONEY;
|
||
|
||
// Depth First search loop for choosing the UTXOs
|
||
for (size_t i = 0; i < TOTAL_TRIES; ++i) {
|
||
// Conditions for starting a backtrack
|
||
bool backtrack = false;
|
||
if (curr_value + curr_available_value < selection_target || // Cannot possibly reach target with the amount remaining in the curr_available_value.
|
||
curr_value > selection_target + cost_of_change || // Selected value is out of range, go back and try other branch
|
||
(curr_waste > best_waste && (utxo_pool.at(0).fee - utxo_pool.at(0).long_term_fee) > 0)) { // Don't select things which we know will be more wasteful if the waste is increasing
|
||
backtrack = true;
|
||
} else if (curr_value >= selection_target) { // Selected value is within range
|
||
curr_waste += (curr_value - selection_target); // This is the excess value which is added to the waste for the below comparison
|
||
// Adding another UTXO after this check could bring the waste down if the long term fee is higher than the current fee.
|
||
// However we are not going to explore that because this optimization for the waste is only done when we have hit our target
|
||
// value. Adding any more UTXOs will be just burning the UTXO; it will go entirely to fees. Thus we aren't going to
|
||
// explore any more UTXOs to avoid burning money like that.
|
||
if (curr_waste <= best_waste) {
|
||
best_selection = curr_selection;
|
||
best_selection.resize(utxo_pool.size());
|
||
best_waste = curr_waste;
|
||
if (best_waste == 0) {
|
||
break;
|
||
}
|
||
}
|
||
curr_waste -= (curr_value - selection_target); // Remove the excess value as we will be selecting different coins now
|
||
backtrack = true;
|
||
}
|
||
|
||
// Backtracking, moving backwards
|
||
if (backtrack) {
|
||
// Walk backwards to find the last included UTXO that still needs to have its omission branch traversed.
|
||
while (!curr_selection.empty() && !curr_selection.back()) {
|
||
curr_selection.pop_back();
|
||
curr_available_value += utxo_pool.at(curr_selection.size()).GetSelectionAmount();
|
||
}
|
||
|
||
if (curr_selection.empty()) { // We have walked back to the first utxo and no branch is untraversed. All solutions searched
|
||
break;
|
||
}
|
||
|
||
// Output was included on previous iterations, try excluding now.
|
||
curr_selection.back() = false;
|
||
OutputGroup& utxo = utxo_pool.at(curr_selection.size() - 1);
|
||
curr_value -= utxo.GetSelectionAmount();
|
||
curr_waste -= utxo.fee - utxo.long_term_fee;
|
||
} else { // Moving forwards, continuing down this branch
|
||
OutputGroup& utxo = utxo_pool.at(curr_selection.size());
|
||
|
||
// Remove this utxo from the curr_available_value utxo amount
|
||
curr_available_value -= utxo.GetSelectionAmount();
|
||
|
||
// Avoid searching a branch if the previous UTXO has the same value and same waste and was excluded. Since the ratio of fee to
|
||
// long term fee is the same, we only need to check if one of those values match in order to know that the waste is the same.
|
||
if (!curr_selection.empty() && !curr_selection.back() &&
|
||
utxo.GetSelectionAmount() == utxo_pool.at(curr_selection.size() - 1).GetSelectionAmount() &&
|
||
utxo.fee == utxo_pool.at(curr_selection.size() - 1).fee) {
|
||
curr_selection.push_back(false);
|
||
} else {
|
||
// Inclusion branch first (Largest First Exploration)
|
||
curr_selection.push_back(true);
|
||
curr_value += utxo.GetSelectionAmount();
|
||
curr_waste += utxo.fee - utxo.long_term_fee;
|
||
}
|
||
}
|
||
}
|
||
|
||
// Check for solution
|
||
if (best_selection.empty()) {
|
||
return std::nullopt;
|
||
}
|
||
|
||
// Set output set
|
||
for (size_t i = 0; i < best_selection.size(); ++i) {
|
||
if (best_selection.at(i)) {
|
||
result.AddInput(utxo_pool.at(i));
|
||
}
|
||
}
|
||
|
||
return result;
|
||
}
|
||
|
||
std::optional<SelectionResult> SelectCoinsSRD(const std::vector<OutputGroup>& utxo_pool, CAmount target_value)
|
||
{
|
||
SelectionResult result(target_value);
|
||
|
||
std::vector<size_t> indexes;
|
||
indexes.resize(utxo_pool.size());
|
||
std::iota(indexes.begin(), indexes.end(), 0);
|
||
Shuffle(indexes.begin(), indexes.end(), FastRandomContext());
|
||
|
||
CAmount selected_eff_value = 0;
|
||
for (const size_t i : indexes) {
|
||
const OutputGroup& group = utxo_pool.at(i);
|
||
Assume(group.GetSelectionAmount() > 0);
|
||
selected_eff_value += group.GetSelectionAmount();
|
||
result.AddInput(group);
|
||
if (selected_eff_value >= target_value) {
|
||
return result;
|
||
}
|
||
}
|
||
return std::nullopt;
|
||
}
|
||
|
||
static void ApproximateBestSubset(const std::vector<OutputGroup>& groups, const CAmount& nTotalLower, const CAmount& nTargetValue,
|
||
std::vector<char>& vfBest, CAmount& nBest, int iterations = 1000)
|
||
{
|
||
std::vector<char> vfIncluded;
|
||
|
||
vfBest.assign(groups.size(), true);
|
||
nBest = nTotalLower;
|
||
|
||
FastRandomContext insecure_rand;
|
||
|
||
for (int nRep = 0; nRep < iterations && nBest != nTargetValue; nRep++)
|
||
{
|
||
vfIncluded.assign(groups.size(), false);
|
||
CAmount nTotal = 0;
|
||
bool fReachedTarget = false;
|
||
for (int nPass = 0; nPass < 2 && !fReachedTarget; nPass++)
|
||
{
|
||
for (unsigned int i = 0; i < groups.size(); i++)
|
||
{
|
||
//The solver here uses a randomized algorithm,
|
||
//the randomness serves no real security purpose but is just
|
||
//needed to prevent degenerate behavior and it is important
|
||
//that the rng is fast. We do not use a constant random sequence,
|
||
//because there may be some privacy improvement by making
|
||
//the selection random.
|
||
if (nPass == 0 ? insecure_rand.randbool() : !vfIncluded[i])
|
||
{
|
||
nTotal += groups[i].GetSelectionAmount();
|
||
vfIncluded[i] = true;
|
||
if (nTotal >= nTargetValue)
|
||
{
|
||
fReachedTarget = true;
|
||
if (nTotal < nBest)
|
||
{
|
||
nBest = nTotal;
|
||
vfBest = vfIncluded;
|
||
}
|
||
nTotal -= groups[i].GetSelectionAmount();
|
||
vfIncluded[i] = false;
|
||
}
|
||
}
|
||
}
|
||
}
|
||
}
|
||
}
|
||
|
||
std::optional<SelectionResult> KnapsackSolver(std::vector<OutputGroup>& groups, const CAmount& nTargetValue)
|
||
{
|
||
SelectionResult result(nTargetValue);
|
||
|
||
// List of values less than target
|
||
std::optional<OutputGroup> lowest_larger;
|
||
std::vector<OutputGroup> applicable_groups;
|
||
CAmount nTotalLower = 0;
|
||
|
||
Shuffle(groups.begin(), groups.end(), FastRandomContext());
|
||
|
||
for (const OutputGroup& group : groups) {
|
||
if (group.GetSelectionAmount() == nTargetValue) {
|
||
result.AddInput(group);
|
||
return result;
|
||
} else if (group.GetSelectionAmount() < nTargetValue + MIN_CHANGE) {
|
||
applicable_groups.push_back(group);
|
||
nTotalLower += group.GetSelectionAmount();
|
||
} else if (!lowest_larger || group.GetSelectionAmount() < lowest_larger->GetSelectionAmount()) {
|
||
lowest_larger = group;
|
||
}
|
||
}
|
||
|
||
if (nTotalLower == nTargetValue) {
|
||
for (const auto& group : applicable_groups) {
|
||
result.AddInput(group);
|
||
}
|
||
return result;
|
||
}
|
||
|
||
if (nTotalLower < nTargetValue) {
|
||
if (!lowest_larger) return std::nullopt;
|
||
result.AddInput(*lowest_larger);
|
||
return result;
|
||
}
|
||
|
||
// Solve subset sum by stochastic approximation
|
||
std::sort(applicable_groups.begin(), applicable_groups.end(), descending);
|
||
std::vector<char> vfBest;
|
||
CAmount nBest;
|
||
|
||
ApproximateBestSubset(applicable_groups, nTotalLower, nTargetValue, vfBest, nBest);
|
||
if (nBest != nTargetValue && nTotalLower >= nTargetValue + MIN_CHANGE) {
|
||
ApproximateBestSubset(applicable_groups, nTotalLower, nTargetValue + MIN_CHANGE, vfBest, nBest);
|
||
}
|
||
|
||
// If we have a bigger coin and (either the stochastic approximation didn't find a good solution,
|
||
// or the next bigger coin is closer), return the bigger coin
|
||
if (lowest_larger &&
|
||
((nBest != nTargetValue && nBest < nTargetValue + MIN_CHANGE) || lowest_larger->GetSelectionAmount() <= nBest)) {
|
||
result.AddInput(*lowest_larger);
|
||
} else {
|
||
for (unsigned int i = 0; i < applicable_groups.size(); i++) {
|
||
if (vfBest[i]) {
|
||
result.AddInput(applicable_groups[i]);
|
||
}
|
||
}
|
||
|
||
if (LogAcceptCategory(BCLog::SELECTCOINS)) {
|
||
std::string log_message{"Coin selection best subset: "};
|
||
for (unsigned int i = 0; i < applicable_groups.size(); i++) {
|
||
if (vfBest[i]) {
|
||
log_message += strprintf("%s ", FormatMoney(applicable_groups[i].m_value));
|
||
}
|
||
}
|
||
LogPrint(BCLog::SELECTCOINS, "%stotal %s\n", log_message, FormatMoney(nBest));
|
||
}
|
||
}
|
||
|
||
return result;
|
||
}
|
||
|
||
/******************************************************************************
|
||
|
||
OutputGroup
|
||
|
||
******************************************************************************/
|
||
|
||
void OutputGroup::Insert(const CInputCoin& output, int depth, bool from_me, size_t ancestors, size_t descendants, bool positive_only) {
|
||
// Compute the effective value first
|
||
const CAmount coin_fee = output.m_input_bytes < 0 ? 0 : m_effective_feerate.GetFee(output.m_input_bytes);
|
||
const CAmount ev = output.txout.nValue - coin_fee;
|
||
|
||
// Filter for positive only here before adding the coin
|
||
if (positive_only && ev <= 0) return;
|
||
|
||
m_outputs.push_back(output);
|
||
CInputCoin& coin = m_outputs.back();
|
||
|
||
coin.m_fee = coin_fee;
|
||
fee += coin.m_fee;
|
||
|
||
coin.m_long_term_fee = coin.m_input_bytes < 0 ? 0 : m_long_term_feerate.GetFee(coin.m_input_bytes);
|
||
long_term_fee += coin.m_long_term_fee;
|
||
|
||
coin.effective_value = ev;
|
||
effective_value += coin.effective_value;
|
||
|
||
m_from_me &= from_me;
|
||
m_value += output.txout.nValue;
|
||
m_depth = std::min(m_depth, depth);
|
||
// ancestors here express the number of ancestors the new coin will end up having, which is
|
||
// the sum, rather than the max; this will overestimate in the cases where multiple inputs
|
||
// have common ancestors
|
||
m_ancestors += ancestors;
|
||
// descendants is the count as seen from the top ancestor, not the descendants as seen from the
|
||
// coin itself; thus, this value is counted as the max, not the sum
|
||
m_descendants = std::max(m_descendants, descendants);
|
||
}
|
||
|
||
bool OutputGroup::EligibleForSpending(const CoinEligibilityFilter& eligibility_filter) const
|
||
{
|
||
return m_depth >= (m_from_me ? eligibility_filter.conf_mine : eligibility_filter.conf_theirs)
|
||
&& m_ancestors <= eligibility_filter.max_ancestors
|
||
&& m_descendants <= eligibility_filter.max_descendants;
|
||
}
|
||
|
||
CAmount OutputGroup::GetSelectionAmount() const
|
||
{
|
||
return m_subtract_fee_outputs ? m_value : effective_value;
|
||
}
|
||
|
||
CAmount GetSelectionWaste(const std::set<CInputCoin>& inputs, CAmount change_cost, CAmount target, bool use_effective_value)
|
||
{
|
||
// This function should not be called with empty inputs as that would mean the selection failed
|
||
assert(!inputs.empty());
|
||
|
||
// Always consider the cost of spending an input now vs in the future.
|
||
CAmount waste = 0;
|
||
CAmount selected_effective_value = 0;
|
||
for (const CInputCoin& coin : inputs) {
|
||
waste += coin.m_fee - coin.m_long_term_fee;
|
||
selected_effective_value += use_effective_value ? coin.effective_value : coin.txout.nValue;
|
||
}
|
||
|
||
if (change_cost) {
|
||
// Consider the cost of making change and spending it in the future
|
||
// If we aren't making change, the caller should've set change_cost to 0
|
||
assert(change_cost > 0);
|
||
waste += change_cost;
|
||
} else {
|
||
// When we are not making change (change_cost == 0), consider the excess we are throwing away to fees
|
||
assert(selected_effective_value >= target);
|
||
waste += selected_effective_value - target;
|
||
}
|
||
|
||
return waste;
|
||
}
|
||
|
||
void SelectionResult::ComputeAndSetWaste(CAmount change_cost)
|
||
{
|
||
m_waste = GetSelectionWaste(m_selected_inputs, change_cost, m_target, m_use_effective);
|
||
}
|
||
|
||
CAmount SelectionResult::GetWaste() const
|
||
{
|
||
Assume(m_waste != std::nullopt);
|
||
return *m_waste;
|
||
}
|
||
|
||
CAmount SelectionResult::GetSelectedValue() const
|
||
{
|
||
return std::accumulate(m_selected_inputs.cbegin(), m_selected_inputs.cend(), CAmount{0}, [](CAmount sum, const auto& coin) { return sum + coin.txout.nValue; });
|
||
}
|
||
|
||
void SelectionResult::Clear()
|
||
{
|
||
m_selected_inputs.clear();
|
||
m_waste.reset();
|
||
}
|
||
|
||
void SelectionResult::AddInput(const OutputGroup& group)
|
||
{
|
||
util::insert(m_selected_inputs, group.m_outputs);
|
||
m_use_effective = !group.m_subtract_fee_outputs;
|
||
}
|
||
|
||
const std::set<CInputCoin>& SelectionResult::GetInputSet() const
|
||
{
|
||
return m_selected_inputs;
|
||
}
|
||
|
||
std::vector<CInputCoin> SelectionResult::GetShuffledInputVector() const
|
||
{
|
||
std::vector<CInputCoin> coins(m_selected_inputs.begin(), m_selected_inputs.end());
|
||
Shuffle(coins.begin(), coins.end(), FastRandomContext());
|
||
return coins;
|
||
}
|
||
|
||
bool SelectionResult::operator<(SelectionResult other) const
|
||
{
|
||
Assume(m_waste != std::nullopt);
|
||
Assume(other.m_waste != std::nullopt);
|
||
// As this operator is only used in std::min_element, we want the result that has more inputs when waste are equal.
|
||
return *m_waste < *other.m_waste || (*m_waste == *other.m_waste && m_selected_inputs.size() > other.m_selected_inputs.size());
|
||
}
|