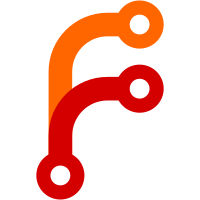
bdf39000b9c Merge bitcoin-core/secp256k1#1223: release: prepare for 0.3.0 b40adf23604 release: prepare for 0.3.0 90b513aadad Merge bitcoin-core/secp256k1#1229: cmake: Rename project to "libsecp256k1" 8be82d43628 cmake: Rename project to "libsecp256k1" ef4f8bd0259 Merge bitcoin-core/secp256k1#1227: readme: Use correct build type in CMake/Windows build instructions 756b61d451d readme: Use correct build type in CMake/Windows build instructions 3295aa149bd Merge bitcoin-core/secp256k1#1225: changelog: Add entry for CMake 92098d84cf7 changelog: Add entry for CMake df323b5c146 Merge bitcoin-core/secp256k1#1113: build: Add CMake-based build system e1eb33724c2 ci: Add "x86_64: Windows (VS 2022)" task 10602b0030e cmake: Export config files 5468d709644 build: Add CMake-based build system 6048e6c03e4 Merge bitcoin-core/secp256k1#1222: Remove redundant checks. eb8749fcd0f Merge bitcoin-core/secp256k1#1221: Update Changelog 5d8f53e3129 Remove redudent checks. 9d1b458d5fb Merge bitcoin-core/secp256k1#1217: Add secp256k1_fe_add_int function d232112fa7e Update Changelog 8962fc95bb0 Merge bitcoin-core/secp256k1#1218: Update overflow check 2ef1c9b3870 Update overflow check 57573187826 Merge bitcoin-core/secp256k1#1212: Prevent dead-store elimination when clearing secrets in examples b081f7e4cbf Add secp256k1_fe_add_int function 5660c137552 prevent optimization in algorithms 09b1d466db7 Merge bitcoin-core/secp256k1#979: Native jacobi symbol algorithm ce3cfc78a60 doc: Describe Jacobi calculation in safegcd_implementation.md 6be01036c8a Add secp256k1_fe_is_square_var function 1de2a01c2b2 Native jacobi symbol algorithm 04c6c1b1816 Make secp256k1_modinv64_det_check_pow2 support abs val 5fffb2c7af5 Make secp256k1_i128_check_pow2 support -(2^n) cbd25559343 Merge bitcoin-core/secp256k1#1209: build: Add SECP256K1_API_VAR to fix importing variables from DLLs 1b21aa51752 Merge bitcoin-core/secp256k1#1078: group: Save a normalize_to_zero in gej_add_ge e4330341bd6 ci: Shutdown wineserver whenever CI script exits 9a5a611a21f build: Suppress stupid MSVC linker warning 739c53b19a2 examples: Extend sig examples by call that uses static context 914276e4d27 build: Add SECP256K1_API_VAR to fix importing variables from DLLs 1cca7c1744b Merge bitcoin-core/secp256k1#1206: build: Add -Wreserved-identifier supported by clang 8c7e0fc1de0 build: Add -Wreserved-identifier supported by clang 8ebe5c52050 Merge bitcoin-core/secp256k1#1201: ci: Do not set git's `user.{email,name}` config options 5596ec5c2cf Merge bitcoin-core/secp256k1#1203: Do not link `bench` and `ctime_tests` to `COMMON_LIB` ef39721ccce Do not link `bench` and `ctime_tests` to `COMMON_LIB` 9b60e3148d8 ci: Do not set git's `user.{email,name}` config options e1817a6f54f Merge bitcoin-core/secp256k1#1199: ci: Minor improvements inspired by Bitcoin Core 1bff2005885 Merge bitcoin-core/secp256k1#1200: Drop no longer used Autoheader macros 9b7d18669dc Drop no longer used Autoheader macros c2415866c7a ci: Don't fetch git history 0ecf3188515 ci: Use remote pull/merge ref instead of local git merge 2b77240b3ba Merge bitcoin-core/secp256k1#1172: benchmarks: fix bench_scalar_split eb6bebaee39 scalar: restrict split_lambda args, improve doc and VERIFY_CHECKs 7f49aa7f2dc ci: add test job with -DVERIFY 620ba3d74be benchmarks: fix bench_scalar_split 5fbff5d348f Merge bitcoin-core/secp256k1#1170: contexts: Forbid destroying, cloning and randomizing the static context 233822d849d Merge bitcoin-core/secp256k1#1195: ctime_tests: improve output when CHECKMEM_RUNNING is not defined ad7433b1409 Merge bitcoin-core/secp256k1#1196: Drop no longer used variables from the build system e39d954f118 tests: Add CHECK_ILLEGAL(_VOID) macros and use in static ctx tests 2cd4e3c0a97 Drop no longer used `SECP_{LIBS,INCLUDE}` variables 613626f94c7 Drop no longer used `SECP_TEST_{LIBS,INCLUDE}` variables 61841fc9ee5 contexts: Forbid randomizing secp256k1_context_static 4b6df5e33e1 contexts: Forbid cloning/destroying secp256k1_context_static b1579cf5fb4 Merge bitcoin-core/secp256k1#1194: Ensure safety of ctz_debruijn implementation. 8f51229e034 ctime_tests: improve output when CHECKMEM_RUNNING is not defined d6ff738d5bb Ensure safety of ctz_debruijn implementation. a01a7d86dc2 Merge bitcoin-core/secp256k1#1192: Switch to exhaustive groups with small B coefficient a7a7bfaf3dc Merge bitcoin-core/secp256k1#1190: Make all non-API functions (except main) static f29a3270923 Merge bitcoin-core/secp256k1#1169: Add support for msan instead of valgrind (for memcheck and ctime test) ff8edf89e2e Merge bitcoin-core/secp256k1#1193: Add `noverify_tests` to `.gitignore` ce60785b265 Introduce SECP256K1_B macro for curve b coefficient 4934aa79958 Switch to exhaustive groups with small B coefficient d4a6b58df74 Add `noverify_tests` to `.gitignore` 88e80722d2a Merge bitcoin-core/secp256k1#1160: Makefile: add `-I$(top_srcdir)/{include,src}` to `CPPFLAGS` for precomputed 0f088ec1126 Rename CTIMETEST -> CTIMETESTS 74b026f05d5 Add runtime checking for DECLASSIFY flag 5e2e6fcfc0e Run ctime test in Linux MSan CI job 18974061a3f Make ctime tests building configurable 5048be17e93 Rename valgrind_ctime_test -> ctime_tests 6eed6c18ded Update error messages to suggest msan as well 8e11f89a685 Add support for msan integration to checkmem.h 8dc64079eb1 Add compile-time error to valgrind_ctime_test 0db05a770eb Abstract interactions with valgrind behind new checkmem.h 4f1a54e41d8 Move valgrind CPPFLAGS into SECP_CONFIG_DEFINES cc3b8a4f404 Merge bitcoin-core/secp256k1#1187: refactor: Rename global variables in tests 9a93f48f502 refactor: Rename STTC to STATIC_CTX in tests 3385a2648d7 refactor: Rename global variables to uppercase in tests e03ef865593 Make all non-API functions (except main) static cbe41ac138b Merge bitcoin-core/secp256k1#1188: tests: Add noverify_tests which is like tests but without VERIFY 203760023c6 tests: Add noverify_tests which is like tests but without VERIFY e862c4af0c5 Makefile: add -I$(top_srcdir)/src to CPPFLAGS for precomputed 0eb3000417f Merge bitcoin-core/secp256k1#1186: tests: Tidy context tests 39e8f0e3d7b refactor: Separate run_context_tests into static vs proper contexts a4a09379b1a tests: Clean up and improve run_context_tests() further fc90bb56956 refactor: Tidy up main() f32a36f620e tests: Don't use global context for context tests ce4f936c4fa tests: Tidy run_context_tests() by extracting functions 18e0db30cb4 tests: Don't recreate global context in scratch space test b19806122e9 tests: Use global copy of secp256k1_context_static instead of clone 2a39ac162e0 Merge bitcoin-core/secp256k1#1185: Drop `SECP_CONFIG_DEFINES` from examples 2f9ca284e2a Drop `SECP_CONFIG_DEFINES` from examples 31ed5386e84 Merge bitcoin-core/secp256k1#1183: Bugfix: pass SECP_CONFIG_DEFINES to bench compilation c0a555b2ae3 Bugfix: pass SECP_CONFIG_DEFINES to bench compilation 01b819a8c7d Merge bitcoin-core/secp256k1#1158: Add a secp256k1_i128_to_u64 function. eacad90f699 Merge bitcoin-core/secp256k1#1171: Change ARG_CHECK_NO_RETURN to ARG_CHECK_VOID which returns (void) 3f57b9f7749 Merge bitcoin-core/secp256k1#1177: Some improvements to the changelog c30b889f17e Clarify that the ABI-incompatible versions are earlier 881fc33d0c1 Consistency in naming of modules 665ba77e793 Merge bitcoin-core/secp256k1#1178: Drop `src/libsecp256k1-config.h` 75d7b7f5bae Merge bitcoin-core/secp256k1#1154: ci: set -u in cirrus.sh to treat unset variables as an error 7a746882013 ci: add missing CFLAGS & CPPFLAGS variable to print_environment c2e0fdadebd ci: set -u in cirrus.sh to treat unset variables as an error 9c5a4d21bbe Do not define unused `HAVE_VALGRIND` macro ad8647f548c Drop no longer relevant files from `.gitignore` b627ba7050b Remove dependency on `src/libsecp256k1-config.h` 9ecf8149a19 Reduce font size in changelog 2dc133a67ff Add more changelog entries ac233e181a5 Add links to diffs to changelog cee8223ef6d Mention semantic versioning in changelog 9a8d65f07f1 Merge bitcoin-core/secp256k1#1174: release cleanup: bump version after 0.2.0 02ebc290f74 release cleanup: bump version after 0.2.0 b6b360efafc doc: improve message of cleanup commit a49e0940ad6 docs: Fix typo 2551cdac903 tests: Fix code formatting c635c1bfd54 Change ARG_CHECK_NO_RETURN to ARG_CHECK_VOID which returns (void) cf66f2357c6 refactor: Add helper function secp256k1_context_is_proper() d2164752053 test secp256k1_i128_to_i64 4bc429019dc Add a secp256k1_i128_to_u64 function. e089eecc1e5 group: Further simply gej_add_ge ac71020ebe0 group: Save a normalize_to_zero in gej_add_ge git-subtree-dir: src/secp256k1 git-subtree-split: bdf39000b9c6a0818e7149ccb500873d079e6e85
34 KiB
The safegcd implementation in libsecp256k1 explained
This document explains the modular inverse and Jacobi symbol implementations in the src/modinv*.h
files.
It is based on the paper
"Fast constant-time gcd computation and modular inversion"
by Daniel J. Bernstein and Bo-Yin Yang. The references below are for the Date: 2019.04.13 version.
The actual implementation is in C of course, but for demonstration purposes Python3 is used here. Most implementation aspects and optimizations are explained, except those that depend on the specific number representation used in the C code.
1. Computing the Greatest Common Divisor (GCD) using divsteps
The algorithm from the paper (section 11), at a very high level, is this:
def gcd(f, g):
"""Compute the GCD of an odd integer f and another integer g."""
assert f & 1 # require f to be odd
delta = 1 # additional state variable
while g != 0:
assert f & 1 # f will be odd in every iteration
if delta > 0 and g & 1:
delta, f, g = 1 - delta, g, (g - f) // 2
elif g & 1:
delta, f, g = 1 + delta, f, (g + f) // 2
else:
delta, f, g = 1 + delta, f, (g ) // 2
return abs(f)
It computes the greatest common divisor of an odd integer f and any integer g. Its inner loop keeps rewriting the variables f and g alongside a state variable δ that starts at 1, until g=0 is reached. At that point, |f| gives the GCD. Each of the transitions in the loop is called a "division step" (referred to as divstep in what follows).
For example, gcd(21, 14) would be computed as:
- Start with δ=1 f=21 g=14
- Take the third branch: δ=2 f=21 g=7
- Take the first branch: δ=-1 f=7 g=-7
- Take the second branch: δ=0 f=7 g=0
- The answer |f| = 7.
Why it works:
- Divsteps can be decomposed into two steps (see paragraph 8.2 in the paper):
- (a) If g is odd, replace (f,g) with (g,g-f) or (f,g+f), resulting in an even g.
- (b) Replace (f,g) with (f,g/2) (where g is guaranteed to be even).
- Neither of those two operations change the GCD:
- For (a), assume gcd(f,g)=c, then it must be the case that f=a c and g=b c for some integers a and b. As (g,g-f)=(b c,(b-a)c) and (f,f+g)=(a c,(a+b)c), the result clearly still has common factor c. Reasoning in the other direction shows that no common factor can be added by doing so either.
- For (b), we know that f is odd, so gcd(f,g) clearly has no factor 2, and we can remove it from g.
- The algorithm will eventually converge to g=0. This is proven in the paper (see theorem G.3).
- It follows that eventually we find a final value f' for which gcd(f,g) = gcd(f',0). As the gcd of f' and 0 is |f'| by definition, that is our answer.
Compared to more traditional GCD algorithms, this one has the property of only ever looking at the low-order bits of the variables to decide the next steps, and being easy to make constant-time (in more low-level languages than Python). The δ parameter is necessary to guide the algorithm towards shrinking the numbers' magnitudes without explicitly needing to look at high order bits.
Properties that will become important later:
- Performing more divsteps than needed is not a problem, as f does not change anymore after g=0.
- Only even numbers are divided by 2. This means that when reasoning about it algebraically we do not need to worry about rounding.
- At every point during the algorithm's execution the next N steps only depend on the bottom N bits of f and g, and on δ.
2. From GCDs to modular inverses
We want an algorithm to compute the inverse a of x modulo M, i.e. the number a such that a x=1 mod M. This inverse only exists if the GCD of x and M is 1, but that is always the case if M is prime and 0 < x < M. In what follows, assume that the modular inverse exists. It turns out this inverse can be computed as a side effect of computing the GCD by keeping track of how the internal variables can be written as linear combinations of the inputs at every step (see the extended Euclidean algorithm). Since the GCD is 1, such an algorithm will compute numbers a and b such that a x + b M = 1*. Taking that expression mod M gives a x mod M = 1, and we see that a is the modular inverse of x mod M.
A similar approach can be used to calculate modular inverses using the divsteps-based GCD algorithm shown above, if the modulus M is odd. To do so, compute gcd(f=M,g=x), while keeping track of extra variables d and e, for which at every step d = f/x (mod M) and e = g/x (mod M). f/x here means the number which multiplied with x gives f mod M. As f and g are initialized to M and x respectively, d and e just start off being 0 (M/x mod M = 0/x mod M = 0) and 1 (x/x mod M = 1).
def div2(M, x):
"""Helper routine to compute x/2 mod M (where M is odd)."""
assert M & 1
if x & 1: # If x is odd, make it even by adding M.
x += M
# x must be even now, so a clean division by 2 is possible.
return x // 2
def modinv(M, x):
"""Compute the inverse of x mod M (given that it exists, and M is odd)."""
assert M & 1
delta, f, g, d, e = 1, M, x, 0, 1
while g != 0:
# Note that while division by two for f and g is only ever done on even inputs, this is
# not true for d and e, so we need the div2 helper function.
if delta > 0 and g & 1:
delta, f, g, d, e = 1 - delta, g, (g - f) // 2, e, div2(M, e - d)
elif g & 1:
delta, f, g, d, e = 1 + delta, f, (g + f) // 2, d, div2(M, e + d)
else:
delta, f, g, d, e = 1 + delta, f, (g ) // 2, d, div2(M, e )
# Verify that the invariants d=f/x mod M, e=g/x mod M are maintained.
assert f % M == (d * x) % M
assert g % M == (e * x) % M
assert f == 1 or f == -1 # |f| is the GCD, it must be 1
# Because of invariant d = f/x (mod M), 1/x = d/f (mod M). As |f|=1, d/f = d*f.
return (d * f) % M
Also note that this approach to track d and e throughout the computation to determine the inverse is different from the paper. There (see paragraph 12.1 in the paper) a transition matrix for the entire computation is determined (see section 3 below) and the inverse is computed from that. The approach here avoids the need for 2x2 matrix multiplications of various sizes, and appears to be faster at the level of optimization we're able to do in C.
3. Batching multiple divsteps
Every divstep can be expressed as a matrix multiplication, applying a transition matrix (1/2 t) to both vectors [f, g] and [d, e] (see paragraph 8.1 in the paper):
t = [ u, v ]
[ q, r ]
[ out_f ] = (1/2 * t) * [ in_f ]
[ out_g ] = [ in_g ]
[ out_d ] = (1/2 * t) * [ in_d ] (mod M)
[ out_e ] [ in_e ]
where (u, v, q, r) is (0, 2, -1, 1), (2, 0, 1, 1), or (2, 0, 0, 1), depending on which branch is taken. As above, the resulting f and g are always integers.
Performing multiple divsteps corresponds to a multiplication with the product of all the individual divsteps' transition matrices. As each transition matrix consists of integers divided by 2, the product of these matrices will consist of integers divided by 2N (see also theorem 9.2 in the paper). These divisions are expensive when updating d and e, so we delay them: we compute the integer coefficients of the combined transition matrix scaled by 2N, and do one division by 2N as a final step:
def divsteps_n_matrix(delta, f, g):
"""Compute delta and transition matrix t after N divsteps (multiplied by 2^N)."""
u, v, q, r = 1, 0, 0, 1 # start with identity matrix
for _ in range(N):
if delta > 0 and g & 1:
delta, f, g, u, v, q, r = 1 - delta, g, (g - f) // 2, 2*q, 2*r, q-u, r-v
elif g & 1:
delta, f, g, u, v, q, r = 1 + delta, f, (g + f) // 2, 2*u, 2*v, q+u, r+v
else:
delta, f, g, u, v, q, r = 1 + delta, f, (g ) // 2, 2*u, 2*v, q , r
return delta, (u, v, q, r)
As the branches in the divsteps are completely determined by the bottom N bits of f and g, this function to compute the transition matrix only needs to see those bottom bits. Furthermore all intermediate results and outputs fit in (N+1)-bit numbers (unsigned for f and g; signed for u, v, q, and r) (see also paragraph 8.3 in the paper). This means that an implementation using 64-bit integers could set N=62 and compute the full transition matrix for 62 steps at once without any big integer arithmetic at all. This is the reason why this algorithm is efficient: it only needs to update the full-size f, g, d, and e numbers once every N steps.
We still need functions to compute:
[ out_f ] = (1/2^N * [ u, v ]) * [ in_f ]
[ out_g ] ( [ q, r ]) [ in_g ]
[ out_d ] = (1/2^N * [ u, v ]) * [ in_d ] (mod M)
[ out_e ] ( [ q, r ]) [ in_e ]
Because the divsteps transformation only ever divides even numbers by two, the result of t [f,g] is always even. When t is a composition of N divsteps, it follows that the resulting f and g will be multiple of 2N, and division by 2N is simply shifting them down:
def update_fg(f, g, t):
"""Multiply matrix t/2^N with [f, g]."""
u, v, q, r = t
cf, cg = u*f + v*g, q*f + r*g
# (t / 2^N) should cleanly apply to [f,g] so the result of t*[f,g] should have N zero
# bottom bits.
assert cf % 2**N == 0
assert cg % 2**N == 0
return cf >> N, cg >> N
The same is not true for d and e, and we need an equivalent of the div2
function for division by 2N mod M.
This is easy if we have precomputed 1/M mod 2N (which always exists for odd M):
def div2n(M, Mi, x):
"""Compute x/2^N mod M, given Mi = 1/M mod 2^N."""
assert (M * Mi) % 2**N == 1
# Find a factor m such that m*M has the same bottom N bits as x. We want:
# (m * M) mod 2^N = x mod 2^N
# <=> m mod 2^N = (x / M) mod 2^N
# <=> m mod 2^N = (x * Mi) mod 2^N
m = (Mi * x) % 2**N
# Subtract that multiple from x, cancelling its bottom N bits.
x -= m * M
# Now a clean division by 2^N is possible.
assert x % 2**N == 0
return (x >> N) % M
def update_de(d, e, t, M, Mi):
"""Multiply matrix t/2^N with [d, e], modulo M."""
u, v, q, r = t
cd, ce = u*d + v*e, q*d + r*e
return div2n(M, Mi, cd), div2n(M, Mi, ce)
With all of those, we can write a version of modinv
that performs N divsteps at once:
def modinv(M, Mi, x):
"""Compute the modular inverse of x mod M, given Mi=1/M mod 2^N."""
assert M & 1
delta, f, g, d, e = 1, M, x, 0, 1
while g != 0:
# Compute the delta and transition matrix t for the next N divsteps (this only needs
# (N+1)-bit signed integer arithmetic).
delta, t = divsteps_n_matrix(delta, f % 2**N, g % 2**N)
# Apply the transition matrix t to [f, g]:
f, g = update_fg(f, g, t)
# Apply the transition matrix t to [d, e]:
d, e = update_de(d, e, t, M, Mi)
return (d * f) % M
This means that in practice we'll always perform a multiple of N divsteps. This is not a problem because once g=0, further divsteps do not affect f, g, d, or e anymore (only δ keeps increasing). For variable time code such excess iterations will be mostly optimized away in later sections.
4. Avoiding modulus operations
So far, there are two places where we compute a remainder of big numbers modulo M: at the end of
div2n
in every update_de
, and at the very end of modinv
after potentially negating d due to the
sign of f. These are relatively expensive operations when done generically.
To deal with the modulus operation in div2n
, we simply stop requiring d and e to be in range
[0,M) all the time. Let's start by inlining div2n
into update_de
, and dropping the modulus
operation at the end:
def update_de(d, e, t, M, Mi):
"""Multiply matrix t/2^N with [d, e] mod M, given Mi=1/M mod 2^N."""
u, v, q, r = t
cd, ce = u*d + v*e, q*d + r*e
# Cancel out bottom N bits of cd and ce.
md = -((Mi * cd) % 2**N)
me = -((Mi * ce) % 2**N)
cd += md * M
ce += me * M
# And cleanly divide by 2**N.
return cd >> N, ce >> N
Let's look at bounds on the ranges of these numbers. It can be shown that |u|+|v| and |q|+|r|
never exceed 2N (see paragraph 8.3 in the paper), and thus a multiplication with t will have
outputs whose absolute values are at most 2N times the maximum absolute input value. In case the
inputs d and e are in (-M,M), which is certainly true for the initial values d=0 and e=1 assuming
M > 1, the multiplication results in numbers in range (-2NM,2NM). Subtracting less than 2N
times M to cancel out N bits brings that up to (-2N+1M,2NM), and
dividing by 2N at the end takes it to (-2M,M). Another application of update_de
would take that
to (-3M,2M), and so forth. This progressive expansion of the variables' ranges can be
counteracted by incrementing d and e by M whenever they're negative:
...
if d < 0:
d += M
if e < 0:
e += M
cd, ce = u*d + v*e, q*d + r*e
# Cancel out bottom N bits of cd and ce.
...
With inputs in (-2M,M), they will first be shifted into range (-M,M), which means that the
output will again be in (-2M,M), and this remains the case regardless of how many update_de
invocations there are. In what follows, we will try to make this more efficient.
Note that increasing d by M is equal to incrementing cd by u M and ce by q M. Similarly, increasing e by M is equal to incrementing cd by v M and ce by r M. So we could instead write:
...
cd, ce = u*d + v*e, q*d + r*e
# Perform the equivalent of incrementing d, e by M when they're negative.
if d < 0:
cd += u*M
ce += q*M
if e < 0:
cd += v*M
ce += r*M
# Cancel out bottom N bits of cd and ce.
md = -((Mi * cd) % 2**N)
me = -((Mi * ce) % 2**N)
cd += md * M
ce += me * M
...
Now note that we have two steps of corrections to cd and ce that add multiples of M: this increment, and the decrement that cancels out bottom bits. The second one depends on the first one, but they can still be efficiently combined by only computing the bottom bits of cd and ce at first, and using that to compute the final md, me values:
def update_de(d, e, t, M, Mi):
"""Multiply matrix t/2^N with [d, e], modulo M."""
u, v, q, r = t
md, me = 0, 0
# Compute what multiples of M to add to cd and ce.
if d < 0:
md += u
me += q
if e < 0:
md += v
me += r
# Compute bottom N bits of t*[d,e] + M*[md,me].
cd, ce = (u*d + v*e + md*M) % 2**N, (q*d + r*e + me*M) % 2**N
# Correct md and me such that the bottom N bits of t*[d,e] + M*[md,me] are zero.
md -= (Mi * cd) % 2**N
me -= (Mi * ce) % 2**N
# Do the full computation.
cd, ce = u*d + v*e + md*M, q*d + r*e + me*M
# And cleanly divide by 2**N.
return cd >> N, ce >> N
One last optimization: we can avoid the md M and me M multiplications in the bottom bits of cd and ce by moving them to the md and me correction:
...
# Compute bottom N bits of t*[d,e].
cd, ce = (u*d + v*e) % 2**N, (q*d + r*e) % 2**N
# Correct md and me such that the bottom N bits of t*[d,e]+M*[md,me] are zero.
# Note that this is not the same as {md = (-Mi * cd) % 2**N} etc. That would also result in N
# zero bottom bits, but isn't guaranteed to be a reduction of [0,2^N) compared to the
# previous md and me values, and thus would violate our bounds analysis.
md -= (Mi*cd + md) % 2**N
me -= (Mi*ce + me) % 2**N
...
The resulting function takes d and e in range (-2M,M) as inputs, and outputs values in the same
range. That also means that the d value at the end of modinv
will be in that range, while we want
a result in [0,M). To do that, we need a normalization function. It's easy to integrate the
conditional negation of d (based on the sign of f) into it as well:
def normalize(sign, v, M):
"""Compute sign*v mod M, where v is in range (-2*M,M); output in [0,M)."""
assert sign == 1 or sign == -1
# v in (-2*M,M)
if v < 0:
v += M
# v in (-M,M). Now multiply v with sign (which can only be 1 or -1).
if sign == -1:
v = -v
# v in (-M,M)
if v < 0:
v += M
# v in [0,M)
return v
And calling it in modinv
is simply:
...
return normalize(f, d, M)
5. Constant-time operation
The primary selling point of the algorithm is fast constant-time operation. What code flow still depends on the input data so far?
- the number of iterations of the while g ≠ 0 loop in
modinv
- the branches inside
divsteps_n_matrix
- the sign checks in
update_de
- the sign checks in
normalize
To make the while loop in modinv
constant time it can be replaced with a constant number of
iterations. The paper proves (Theorem 11.2) that 741 divsteps are sufficient for any 256-bit
inputs, and safegcd-bounds shows that the slightly better bound 724 is
sufficient even. Given that every loop iteration performs N divsteps, it will run a total of
⌈724/N⌉ times.
To deal with the branches in divsteps_n_matrix
we will replace them with constant-time bitwise
operations (and hope the C compiler isn't smart enough to turn them back into branches; see
ctime_tests.c
for automated tests that this isn't the case). To do so, observe that a
divstep can be written instead as (compare to the inner loop of gcd
in section 1).
x = -f if delta > 0 else f # set x equal to (input) -f or f
if g & 1:
g += x # set g to (input) g-f or g+f
if delta > 0:
delta = -delta
f += g # set f to (input) g (note that g was set to g-f before)
delta += 1
g >>= 1
To convert the above to bitwise operations, we rely on a trick to negate conditionally: per the definition of negative numbers in two's complement, (-v == ~v + 1) holds for every number v. As -1 in two's complement is all 1 bits, bitflipping can be expressed as xor with -1. It follows that -v == (v ^ -1) - (-1). Thus, if we have a variable c that takes on values 0 or -1, then (v ^ c) - c is v if c=0 and -v if c=-1.
Using this we can write:
x = -f if delta > 0 else f
in constant-time form as:
c1 = (-delta) >> 63
# Conditionally negate f based on c1:
x = (f ^ c1) - c1
To use that trick, we need a helper mask variable c1 that resolves the condition δ>0 to -1
(if true) or 0 (if false). We compute c1 using right shifting, which is equivalent to dividing by
the specified power of 2 and rounding down (in Python, and also in C under the assumption of a typical two's complement system; see
assumptions.h
for tests that this is the case). Right shifting by 63 thus maps all
numbers in range [-263,0) to -1, and numbers in range [0,263) to 0.
Using the facts that x&0=0 and x&(-1)=x (on two's complement systems again), we can write:
if g & 1:
g += x
as:
# Compute c2=0 if g is even and c2=-1 if g is odd.
c2 = -(g & 1)
# This masks out x if g is even, and leaves x be if g is odd.
g += x & c2
Using the conditional negation trick again we can write:
if g & 1:
if delta > 0:
delta = -delta
as:
# Compute c3=-1 if g is odd and delta>0, and 0 otherwise.
c3 = c1 & c2
# Conditionally negate delta based on c3:
delta = (delta ^ c3) - c3
Finally:
if g & 1:
if delta > 0:
f += g
becomes:
f += g & c3
It turns out that this can be implemented more efficiently by applying the substitution η=-δ. In this representation, negating δ corresponds to negating η, and incrementing δ corresponds to decrementing η. This allows us to remove the negation in the c1 computation:
# Compute a mask c1 for eta < 0, and compute the conditional negation x of f:
c1 = eta >> 63
x = (f ^ c1) - c1
# Compute a mask c2 for odd g, and conditionally add x to g:
c2 = -(g & 1)
g += x & c2
# Compute a mask c for (eta < 0) and odd (input) g, and use it to conditionally negate eta,
# and add g to f:
c3 = c1 & c2
eta = (eta ^ c3) - c3
f += g & c3
# Incrementing delta corresponds to decrementing eta.
eta -= 1
g >>= 1
A variant of divsteps with better worst-case performance can be used instead: starting δ at 1/2 instead of 1. This reduces the worst case number of iterations to 590 for 256-bit inputs (which can be shown using convex hull analysis). In this case, the substitution ζ=-(δ+1/2) is used instead to keep the variable integral. Incrementing δ by 1 still translates to decrementing ζ by 1, but negating δ now corresponds to going from ζ to -(ζ+1), or ~ζ. Doing that conditionally based on c3 is simply:
...
c3 = c1 & c2
zeta ^= c3
...
By replacing the loop in divsteps_n_matrix
with a variant of the divstep code above (extended to
also apply all f operations to u, v and all g operations to q, r), a constant-time version of
divsteps_n_matrix
is obtained. The full code will be in section 7.
These bit fiddling tricks can also be used to make the conditional negations and additions in
update_de
and normalize
constant-time.
6. Variable-time optimizations
In section 5, we modified the divsteps_n_matrix
function (and a few others) to be constant time.
Constant time operations are only necessary when computing modular inverses of secret data. In
other cases, it slows down calculations unnecessarily. In this section, we will construct a
faster non-constant time divsteps_n_matrix
function.
To do so, first consider yet another way of writing the inner loop of divstep operations in
gcd
from section 1. This decomposition is also explained in the paper in section 8.2. We use
the original version with initial δ=1 and η=-δ here.
for _ in range(N):
if g & 1 and eta < 0:
eta, f, g = -eta, g, -f
if g & 1:
g += f
eta -= 1
g >>= 1
Whenever g is even, the loop only shifts g down and decreases η. When g ends in multiple zero
bits, these iterations can be consolidated into one step. This requires counting the bottom zero
bits efficiently, which is possible on most platforms; it is abstracted here as the function
count_trailing_zeros
.
def count_trailing_zeros(v):
"""
When v is zero, consider all N zero bits as "trailing".
For a non-zero value v, find z such that v=(d<<z) for some odd d.
"""
if v == 0:
return N
else:
return (v & -v).bit_length() - 1
i = N # divsteps left to do
while True:
# Get rid of all bottom zeros at once. In the first iteration, g may be odd and the following
# lines have no effect (until "if eta < 0").
zeros = min(i, count_trailing_zeros(g))
eta -= zeros
g >>= zeros
i -= zeros
if i == 0:
break
# We know g is odd now
if eta < 0:
eta, f, g = -eta, g, -f
g += f
# g is even now, and the eta decrement and g shift will happen in the next loop.
We can now remove multiple bottom 0 bits from g at once, but still need a full iteration whenever there is a bottom 1 bit. In what follows, we will get rid of multiple 1 bits simultaneously as well.
Observe that as long as η ≥ 0, the loop does not modify f. Instead, it cancels out bottom bits of g and shifts them out, and decreases η and i accordingly - interrupting only when η becomes negative, or when i reaches 0. Combined, this is equivalent to adding a multiple of f to g to cancel out multiple bottom bits, and then shifting them out.
It is easy to find what that multiple is: we want a number w such that g+w f has a few bottom
zero bits. If that number of bits is L, we want g+w f mod 2L = 0, or w = -g/f mod 2L. Since f
is odd, such a w exists for any L. L cannot be more than i steps (as we'd finish the loop before
doing more) or more than η+1 steps (as we'd run eta, f, g = -eta, g, -f
at that point), but
apart from that, we're only limited by the complexity of computing w.
This code demonstrates how to cancel up to 4 bits per step:
NEGINV16 = [15, 5, 3, 9, 7, 13, 11, 1] # NEGINV16[n//2] = (-n)^-1 mod 16, for odd n
i = N
while True:
zeros = min(i, count_trailing_zeros(g))
eta -= zeros
g >>= zeros
i -= zeros
if i == 0:
break
# We know g is odd now
if eta < 0:
eta, f, g = -eta, g, -f
# Compute limit on number of bits to cancel
limit = min(min(eta + 1, i), 4)
# Compute w = -g/f mod 2**limit, using the table value for -1/f mod 2**4. Note that f is
# always odd, so its inverse modulo a power of two always exists.
w = (g * NEGINV16[(f & 15) // 2]) % (2**limit)
# As w = -g/f mod (2**limit), g+w*f mod 2**limit = 0 mod 2**limit.
g += w * f
assert g % (2**limit) == 0
# The next iteration will now shift out at least limit bottom zero bits from g.
By using a bigger table more bits can be cancelled at once. The table can also be implemented as a formula. Several formulas are known for computing modular inverses modulo powers of two; some can be found in Hacker's Delight second edition by Henry S. Warren, Jr. pages 245-247. Here we need the negated modular inverse, which is a simple transformation of those:
- Instead of a 3-bit table:
- -f or f ^ 6
- Instead of a 4-bit table:
- 1 - f(f + 1)
- -(f + (((f + 1) & 4) << 1))
- For larger tables the following technique can be used: if w=-1/f mod 2L, then w(w f+2) is
-1/f mod 22L. This allows extending the previous formulas (or tables). In particular we
have this 6-bit function (based on the 3-bit function above):
- f(f2 - 2)
This loop, again extended to also handle u, v, q, and r alongside f and g, placed in
divsteps_n_matrix
, gives a significantly faster, but non-constant time version.
7. Final Python version
All together we need the following functions:
- A way to compute the transition matrix in constant time, using the
divsteps_n_matrix
function from section 2, but with its loop replaced by a variant of the constant-time divstep from section 5, extended to handle u, v, q, r:
def divsteps_n_matrix(zeta, f, g):
"""Compute zeta and transition matrix t after N divsteps (multiplied by 2^N)."""
u, v, q, r = 1, 0, 0, 1 # start with identity matrix
for _ in range(N):
c1 = zeta >> 63
# Compute x, y, z as conditionally-negated versions of f, u, v.
x, y, z = (f ^ c1) - c1, (u ^ c1) - c1, (v ^ c1) - c1
c2 = -(g & 1)
# Conditionally add x, y, z to g, q, r.
g, q, r = g + (x & c2), q + (y & c2), r + (z & c2)
c1 &= c2 # reusing c1 here for the earlier c3 variable
zeta = (zeta ^ c1) - 1 # inlining the unconditional zeta decrement here
# Conditionally add g, q, r to f, u, v.
f, u, v = f + (g & c1), u + (q & c1), v + (r & c1)
# When shifting g down, don't shift q, r, as we construct a transition matrix multiplied
# by 2^N. Instead, shift f's coefficients u and v up.
g, u, v = g >> 1, u << 1, v << 1
return zeta, (u, v, q, r)
- The functions to update f and g, and d and e, from section 2 and section 4, with the constant-time
changes to
update_de
from section 5:
def update_fg(f, g, t):
"""Multiply matrix t/2^N with [f, g]."""
u, v, q, r = t
cf, cg = u*f + v*g, q*f + r*g
return cf >> N, cg >> N
def update_de(d, e, t, M, Mi):
"""Multiply matrix t/2^N with [d, e], modulo M."""
u, v, q, r = t
d_sign, e_sign = d >> 257, e >> 257
md, me = (u & d_sign) + (v & e_sign), (q & d_sign) + (r & e_sign)
cd, ce = (u*d + v*e) % 2**N, (q*d + r*e) % 2**N
md -= (Mi*cd + md) % 2**N
me -= (Mi*ce + me) % 2**N
cd, ce = u*d + v*e + M*md, q*d + r*e + M*me
return cd >> N, ce >> N
- The
normalize
function from section 4, made constant time as well:
def normalize(sign, v, M):
"""Compute sign*v mod M, where v in (-2*M,M); output in [0,M)."""
v_sign = v >> 257
# Conditionally add M to v.
v += M & v_sign
c = (sign - 1) >> 1
# Conditionally negate v.
v = (v ^ c) - c
v_sign = v >> 257
# Conditionally add M to v again.
v += M & v_sign
return v
- And finally the
modinv
function too, adapted to use ζ instead of δ, and using the fixed iteration count from section 5:
def modinv(M, Mi, x):
"""Compute the modular inverse of x mod M, given Mi=1/M mod 2^N."""
zeta, f, g, d, e = -1, M, x, 0, 1
for _ in range((590 + N - 1) // N):
zeta, t = divsteps_n_matrix(zeta, f % 2**N, g % 2**N)
f, g = update_fg(f, g, t)
d, e = update_de(d, e, t, M, Mi)
return normalize(f, d, M)
- To get a variable time version, replace the
divsteps_n_matrix
function with one that uses the divsteps loop from section 5, and amodinv
version that calls it without the fixed iteration count:
NEGINV16 = [15, 5, 3, 9, 7, 13, 11, 1] # NEGINV16[n//2] = (-n)^-1 mod 16, for odd n
def divsteps_n_matrix_var(eta, f, g):
"""Compute eta and transition matrix t after N divsteps (multiplied by 2^N)."""
u, v, q, r = 1, 0, 0, 1
i = N
while True:
zeros = min(i, count_trailing_zeros(g))
eta, i = eta - zeros, i - zeros
g, u, v = g >> zeros, u << zeros, v << zeros
if i == 0:
break
if eta < 0:
eta, f, u, v, g, q, r = -eta, g, q, r, -f, -u, -v
limit = min(min(eta + 1, i), 4)
w = (g * NEGINV16[(f & 15) // 2]) % (2**limit)
g, q, r = g + w*f, q + w*u, r + w*v
return eta, (u, v, q, r)
def modinv_var(M, Mi, x):
"""Compute the modular inverse of x mod M, given Mi = 1/M mod 2^N."""
eta, f, g, d, e = -1, M, x, 0, 1
while g != 0:
eta, t = divsteps_n_matrix_var(eta, f % 2**N, g % 2**N)
f, g = update_fg(f, g, t)
d, e = update_de(d, e, t, M, Mi)
return normalize(f, d, Mi)
8. From GCDs to Jacobi symbol
We can also use a similar approach to calculate Jacobi symbol (x | M) by keeping track of an extra variable j, for which at every step (x | M) = j (g | f). As we update f and g, we make corresponding updates to j using properties of the Jacobi symbol:
- ((g/2) | f) is either (g | f) or -(g | f), depending on the value of f mod 8 (negating if it's 3 or 5).
- (f | g) is either (g | f) or -(g | f), depending on f mod 4 and g mod 4 (negating if both are 3).
These updates depend only on the values of f and g modulo 4 or 8, and can thus be applied very quickly, as long as we keep track of a few additional bits of f and g. Overall, this calculation is slightly simpler than the one for the modular inverse because we no longer need to keep track of d and e.
However, one difficulty of this approach is that the Jacobi symbol (a | n) is only defined for positive odd integers n, whereas in the original safegcd algorithm, f, g can take negative values. We resolve this by using the following modified steps:
# Before
if delta > 0 and g & 1:
delta, f, g = 1 - delta, g, (g - f) // 2
# After
if delta > 0 and g & 1:
delta, f, g = 1 - delta, g, (g + f) // 2
The algorithm is still correct, since the changed divstep, called a "posdivstep" (see section 8.4 and E.5 in the paper) preserves gcd(f, g). However, there's no proof that the modified algorithm will converge. The justification for posdivsteps is completely empirical: in practice, it appears that the vast majority of nonzero inputs converge to f=g=gcd(f0, g0) in a number of steps proportional to their logarithm.
Note that:
- We require inputs to satisfy gcd(x, M) = 1, as otherwise f=1 is not reached.
- We require inputs x &neq; 0, because applying posdivstep with g=0 has no effect.
- We need to update the termination condition from g=0 to f=1.
We account for the possibility of nonconvergence by only performing a bounded number of posdivsteps, and then falling back to square-root based Jacobi calculation if a solution has not yet been found.
The optimizations in sections 3-7 above are described in the context of the original divsteps, but in the C implementation we also adapt most of them (not including "avoiding modulus operations", since it's not necessary to track d, e, and "constant-time operation", since we never calculate Jacobi symbols for secret data) to the posdivsteps version.